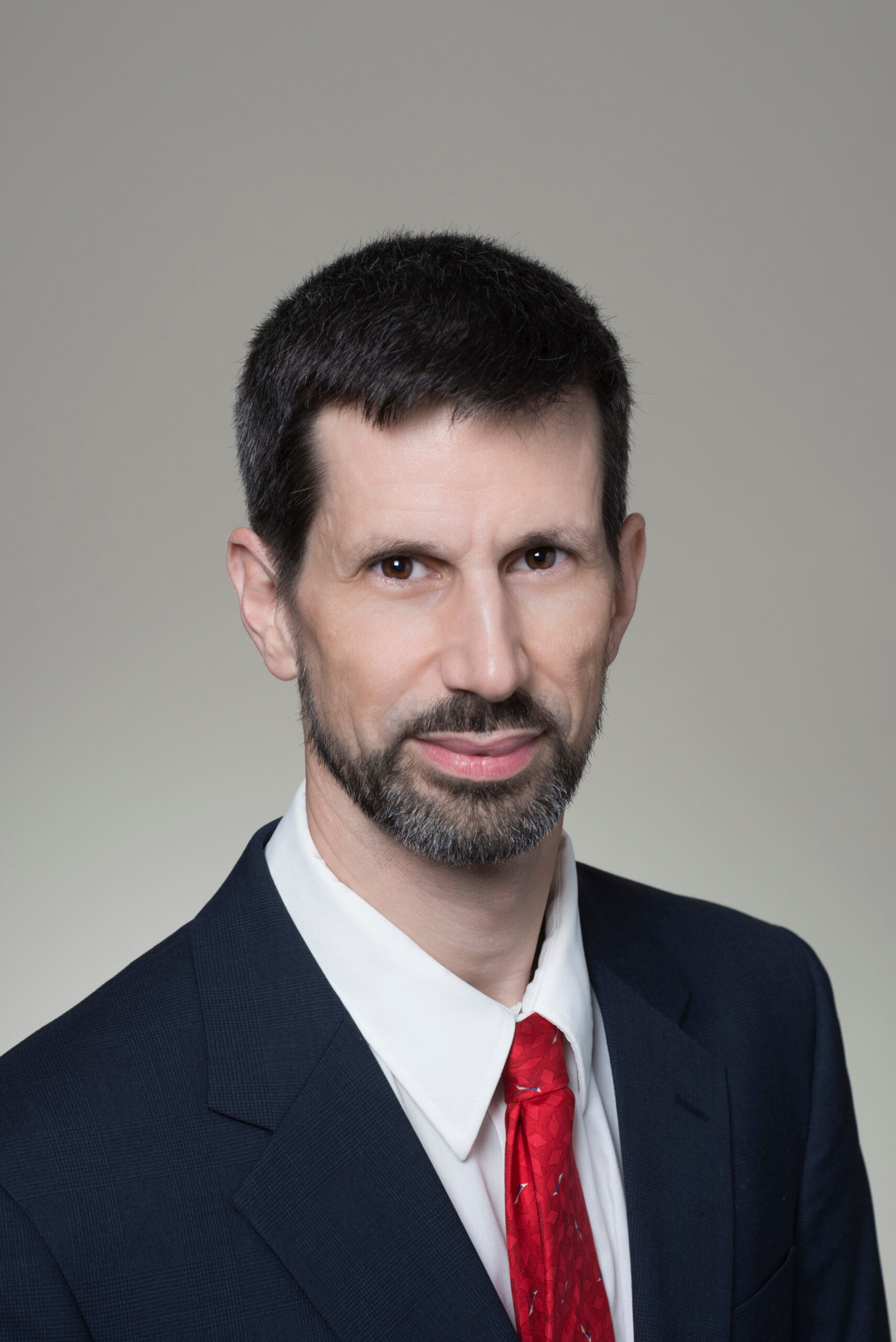
Do Models Generate Realistic Simulations?
This event has passed. View the seminar recording here:
Prof. Timothy Delsole
Department of Atmospheric, Oceanic, and Earth Sciences, &
Center for Ocean-Land-Atmospheric Sciences
George Mason University
Monday, December 6, 2021, 2 PM ET
Abstract:
After a new climate model is constructed, a natural question is whether it generates realistic simulations. By “realistic,” I mean that the statistics over many years are realistic (as opposed to predicting the detailed weather pattern on a given day). Past approaches to answering this question often neglect correlations in space and time. In this talk, I discuss a new method for answering this question that accounts for correlations in space and time. The basic idea is to fit each multivariate time series to a Vector Autoregressive (VAR) Model, and then test the hypothesis that the parameters of the two models are equal. Because a VAR model uniquely specifies the autocorrelation function and the power spectra, equality of VAR models implies equality of autocorrelation functions and equality of power spectra. A first-order VAR model is equivalent to a Linear Inverse Model (LIM), hence the test can be used to test differences in LIMs too. The new test leads to a statistic that measures the dissimilarity between VAR processes and can be used to rank models based on their “closeness” to the VAR process inferred from observations. This test is applied to decide if climate models generate realistic internal variability of annual mean North Atlantic Sea Surface Temperature (NASST). Only a few climate models out of three dozen are found to generate internal variability consistent with observations. In fact, climate models differ not only from observations, but also from each other, unless they come from the same modeling center. Other studies show that models differ from observations also in terms of the response to external forcing. Together, these discrepancies imply that current climate models do not satisfactorily simulate variability in the North Atlantic.
Biosketch:
Timothy DelSole is a full professor in the Department of Atmospheric, Oceanic, and Earth Sciences at George Mason University, and a senior research scientist at the Center for Ocean-Land-Atmosphere Studies. His research focuses on the extent to which weather and climate changes can be predicted on time scales from weeks to years. He has been a contributing author and reviewer for the Intergovernmental Panel on Climate Change. He is the author of over 120 peer-reviewed scientific papers and a new book to be published this month (December 2021) titled “Statistical Methods for Climate Scientists.” He currently serves as co-Chief Editor of Journal of Climate.
The new book debut and one free copy giveaway
We are excited to welcome Prof. Delsole as he debuts a new book, “Statistical Methods for Climate Scientists”, published by the Cambridge University Press in December 2021. To be eligible, you need to sign up to the giveaway using the link below and attend the live webinar. To sign up and read the other specifications required to win, visit: https://go.umd.edu/delsolebook
Statistical Methods for Climate Scientists is a comprehensive introduction to the most commonly used statistical methods relevant in atmospheric, oceanic and climate sciences. Each method is described step-by-step using plain language, and illustrated with concrete examples, with relevant statistical and scientific concepts explained as needed. Particular attention is paid to nuances and pitfalls, with sufficient detail to enable the reader to write relevant code. Topics covered include hypothesis testing, time series analysis, linear regression, data assimilation, extreme value analysis, Principal Component Analysis, Canonical Correlation Analysis, Predictable Component Analysis, and Covariance Discriminant Analysis. The specific statistical challenges that arise in climate applications are also discussed, including model selection problems associated with Canonical Correlation Analysis, Predictable Component Analysis, and Covariance Discriminant Analysis. Requiring no previous background in statistics, this is a highly accessible textbook and reference for students and early-career researchers in the climate sciences.
Webinar:
Webinar: https://go.umd.edu/delsolewebinar
Event site: https://go.umd.edu/delsole
Webinar number: 2621 017 5468
Webinar password: essic
To join the audio conference only:
US Toll: +1-415-655-0002
Global call-in numbers
For IT assistance:
Cazzy Medley: cazzy@umd.edu
Resources:
Seminar schedule & archive: https://go.umd.edu/essicseminar
Seminar Google calendar: https://go.umd.edu/essicseminarcalendar
Seminar recordings on Youtube: https://www.youtube.com/user/ESSICUMD